Dr Brendan Palmer
In this series of short posts we have asked colleagues and peers for their three top tips for effective data management and stewardship. Overtime we hope to build a collection of advice from across the research data ecosystem from data stewards, IT professionals, researchers and all those working with data. Here we share the first in the series from Dr. Brendan Palmer, Senior Manager of Data Services at the Statistics, Data & Analysis Unit, Clinical Research Facility – University College Cork based on his years’ experiences of working with health research data.
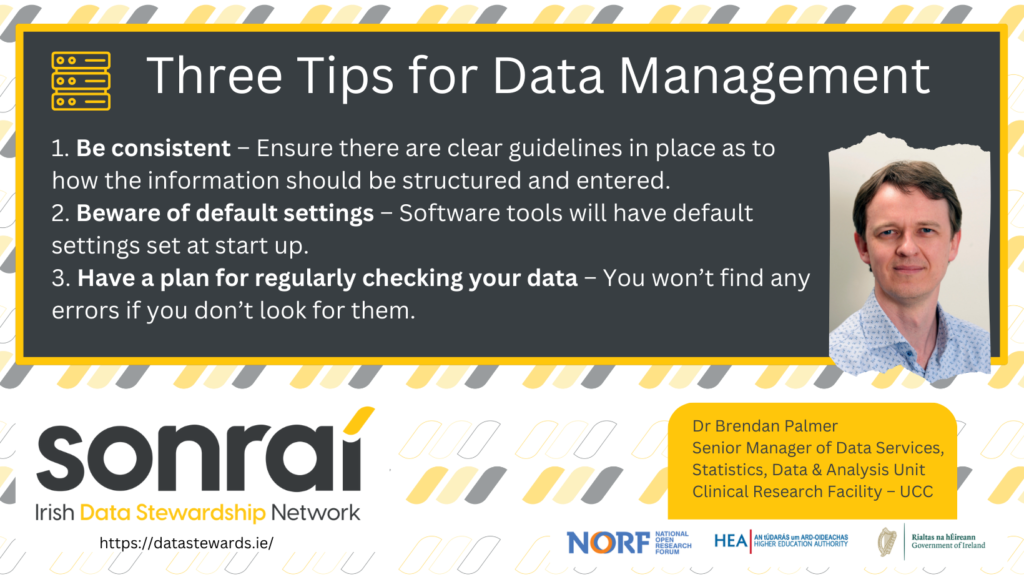
Number 1.
Be consistent – When capturing information, ensure that there are clear guidelines in place as to how the information should be structured and entered.
You may have many staff from different backgrounds tasked with data entry.
Dates are the most obvious example.
-
-
- 31st December 2023 (Format: Day Month Year)
- 31-Dec-2023 (Format: Day-Month-Year)
- 31-12-2023 (Format: DD-MM-YYYY)
- 31-12-23 (Format: DD-MM-YY)
- 31/12/23 (Format: DD/MM/YY)
- 12-31-23 (Format: MM-DD-YY)
-
While the same information is provided, the formats applied are inconsistent and downstream processing of this information will pose unnecessary challenges.
Number 2.
Beware of default settings – Software tools will have default settings in place at start up. You should familiarise yourself with these and adjust as required.
For example, recent releases of Microsoft Office tools are automatically set to “Autosave” once the original document is saved to your system.
This is problematic if you are using MS Excel for data capture as inadvertent changes to the database will be saved without your knowledge. In this instance, Autosave should be deactivated. At least then, a pop-up will appear when you close out of the program asking if you would like the changes to be saved.
Number 3.
Have a plan for regularly checking the data for errors – You won’t find any errors if you aren’t actively looking for them.
Project supervisors, journal reviewers etc. will not have the time (or access) to check the data supporting your findings for errors.
It is inevitable that data files contain errors. Some might be minor, others may lead to spurious findings and conclusions. You should have some process in place to check for errors at regular intervals, be it visualisation, tabulation or review of source documentation against the main database.
If you find our 3 tips from the experts series useful or would like to submit your own tips please do get in contact via our membership page.